Better Battery Design Using AI
Next-generation batteries with high energy density, quick charging capacity, and long lifetimes are needed to enable the energy transition and slow the effects of climate change. The materials challenges involved in battery development are complex and span multiple scales from the molecular and chemical composition of the ceramics used in lithium-ion battery cathodes to the coatings on the cooling channels inside battery packs. This paper explores how AI can be used to reach achieve superior battery performance targets quickly, through material development, battery configuration optimization, and improved battery testing.
Case study: Cathode Configuration optimization
In this paper, we describe a case study a for replacing N-methyl pyrrolidinone (NMP) as a cathode slurry solvent using AI-driven R&D workflows based on regulatory and sustainability concerns around NMP.
We walk through the primary steps to applying an AI-driven workflow including:
- Curating and structuring a product development dataset
- Building a graphical AI model that incorporates scientific domain knowledge
- Training the AI model on the structured battery dataset
- Evaluating model performance
- Defining a search space to constrain ingredients, labels, and processing parameters
- Combining steps 1-5 in an an inverse design workflow to identify novel cathode slurry solvents that hit performance and sustainability targets
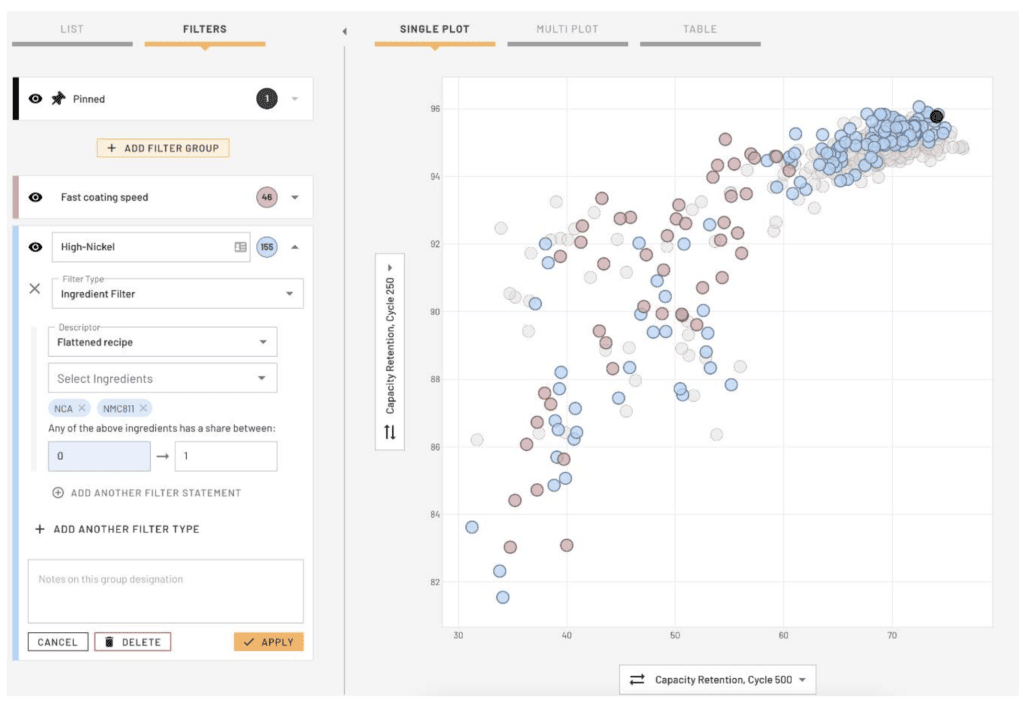
Fill out the form to read the paper.