We were asked to write a perspective paper for the Journal of Data-centric Engineering on this topic. You can read it here: https://www.doi.org/10.1017/dce.2021.19. The following is a more digestible version.
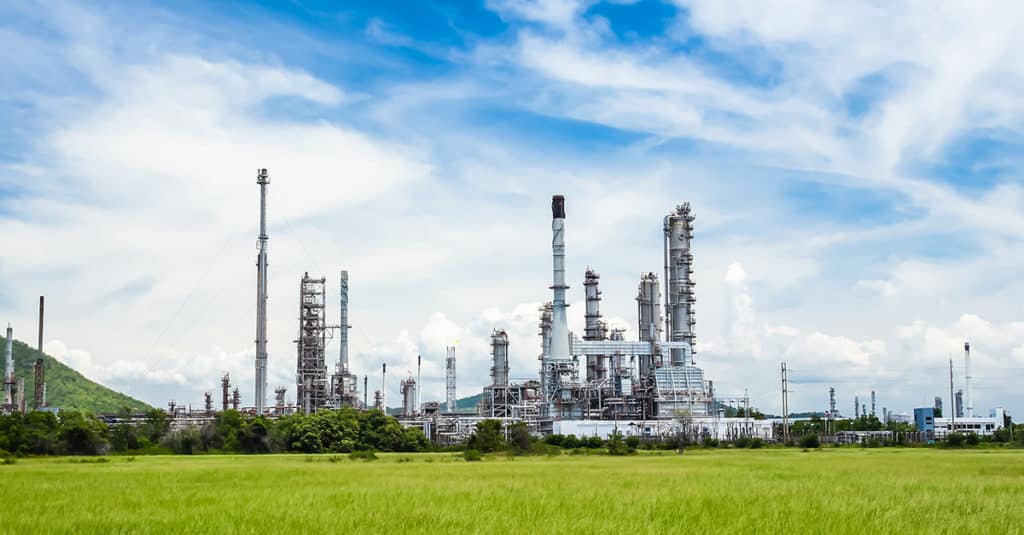
Materials innovation is essential for sustainability
The production of materials and chemicals is estimated to cause around a quarter of greenhouse gases.[1] Moving to biofeedstocks, enabling a circular economy, and increasing the efficiency of material production are all therefore important in meeting global carbon neutral targets. Materials are also part of products that are needed to help us all live more sustainably – contributing to decarbonization of the grid, electromobility, carbon capture, and so on. The development of transformative technologies for mitigating our global environmental and technological challenges will require significant innovation in the design, development, and manufacturing of advanced materials and chemicals.
[1] https://www.nature.com/articles/s41561-021-00690-8
Materials innovation takes too long
Developing new materials and getting them qualified to use in critical applications still takes too long. Twenty years from discovery to market is common.[2] To achieve this innovation faster than what is possible by traditional human intuition–guided scientific methods, we must transition to a materials informatics–centered paradigm. Synergies between data science, materials science, and artificial intelligence can be leveraged to enable transformative, data-driven discoveries through the use of predictive models and digital twins.
Materials Informatics is increasingly adopted by industry
Materials informatics (MI) is experiencing rapidly increasing use across the materials and chemicals industries, with cutting-edge companies leading the way. Common MI applications encountered in materials and chemicals industries today include:
- The discovery of new materials that have specific target properties
- Optimization of the composition or processing parameters of existing materials
- Identification of formulations that simultaneously meet performance, cost, and sustainability criteria
- Identification of the most informative experiments to perform under budgetary requirements or other constraints
You can read case studies from companies like Panasonic and Showa Denko here.[3] However, broad adoption in smaller companies and academia is hindered by barriers such as skill gaps, cultural resistance, and data sparsity.
[3] https://citrine.io/success/case-studies/
Education is needed
MI software platforms are available and are maturing to require less expertise in data science to use them; however, a basic understanding of machine learning and data engineering is necessary to get the most out of the technology. This means that if all materials and chemicals researchers across industry and academia are to accerlerate their reseach, both undergraduate and continuous professional development courses in materials informatics need to be widely available.
This educational process also needs to reach skeptics and the misinformed who do not yet realize the power of MI or who worry that it will take over their jobs. It is important to emphasize that machine learning is not necessarily a black box, and that the domain knowledge of experts is what enables machine learning to be used on small, sparse datasets such as those found in materials and chemicals.
Future public materials data should be FAIR
Materials and chemicals data is expensive to acquire, with a single data point sometimes taking months and costing tens of thousands of pounds. It is also very context specific. A research team in the next lab may not be able to interpret and reuse data if the exact testing circumstances are not included in metadata. In general, the more high-quality data available, the better machine learning models perform. So it is important that future materials data is stored according to FAIR[4] principles, something that can be encouraged by research funding bodies. Just as with the human genome project, access to high-quality materials data could have a transformative catalysing effect on materials research.
[4] https://www.force11.org/group/fairgroup/fairprinciples
Read the paper to hear about best practices
In this perspective paper[5] we discuss the importance of materials informatics for accelerating technological innovation, describe current barriers and examples of good practices, and offer suggestions for how researchers, funding agencies, and educational institutions can help accelerate the adoption of urgently needed informatics-based toolsets for science in the 21st century.
[5] https://www.doi.org/10.1017/dce.2021.19