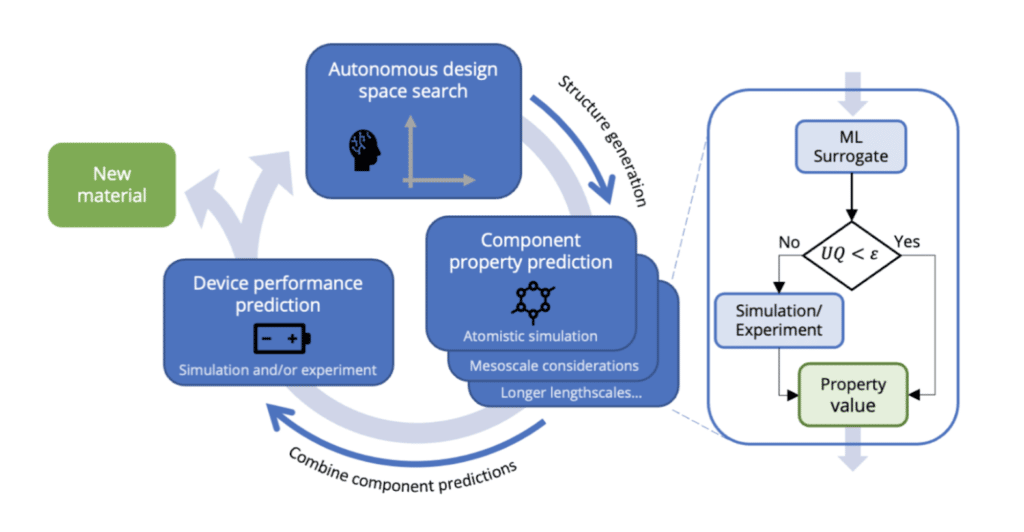
Large-scale electrification is crucial to tackling key climate challenges. Two critical areas where electrification can have an outsized impact are energy storage and chemical manufacturing. For example, newer batteries with improved energy and power capacity are necessary for the wider adoption of electric transportation. Similarly, replacing traditional fossil fuel-based manufacturing of ammonia for fertilizers with electrocatalysts, by itself, can save 5% of all global energy production. To realize the promise of electrochemistry in these areas, we urgently need new, performant materials.
Accelerating new materials discovery
Several efforts are ongoing worldwide to discover new materials to address key energy challenges such as the ones mentioned above. In particular, the past few years have seen a gradual shift towards the closed-loop automation of materials discovery workflows, often with experimental design driven by sequential learning, to accelerate this search for new materials. But how much can a combination of sequential learning and closed-loop automation really accelerate materials discovery?
Collaborating to benchmark acceleration
To answer this question, Citrine collaborated with teams from Carnegie Mellon University, Massachusetts Institute of Technology, and Julia Computing as part of a US Department of Energy ARPA-E initiative called DIFFERENTIATE. The team built closed-loop computational frameworks to discover new catalysts for electrochemical nitrogen reduction as well as new electrolytes for lithium-ion batteries. The speedups from each component in a closed-loop workflow (e.g., from task automation, replacing time-consuming physics-based simulations with machine learning surrogates, using sequential learning to choose candidates to evaluate in each iteration) were carefully benchmarked against traditional approaches.
Accelerating Materials Discovery by 10-25x
Our work shows that a fully-automated closed-loop framework driven by sequential learning can accelerate the discovery of materials by up to 10-25x (or a reduction in design time by 90-95%) when compared to traditional approaches. We show that such closed-loop frameworks can lead to enormous improvement in researcher productivity in addition to reducing overall project costs. Overall, these findings present a clear value proposition for investing in closed-loop frameworks and sequential learning in materials discovery and design enterprises.
batteries (below).
Read More
A couple of manuscript preprints that discuss our findings in greater detail can be found here:
- https://arxiv.org/pdf/2011.04426.pdf (accepted for publication in MRS Bulletin)
- https://arxiv.org/pdf/2211.10533.pdf
Here are some videos that demonstrate the closed-loop frameworks in action:
- Catalysts (Phase I): https://www.youtube.com/watch?v=q7646pX34GU
- Battery electrolytes (Phase II): https://www.youtube.com/watch?v=iGYtjBzF9YE