As organizations embrace the concept of the Digital Twin as a way to increase development agility, it becomes even more important to understand the size of the “error bars” on each simulation.
Density Functional Theory
In the materials world, physics-based simulations such as density functional theory (DFT) are used to calculate material properties. Over the last decade high-throughput DFT has been used to create large, open databases with properties of hundreds of thousands of materials. These databases are now routinely used to build models that predict materials properties and behavior, in order to minimize risks throughout the lifecycle of a product.
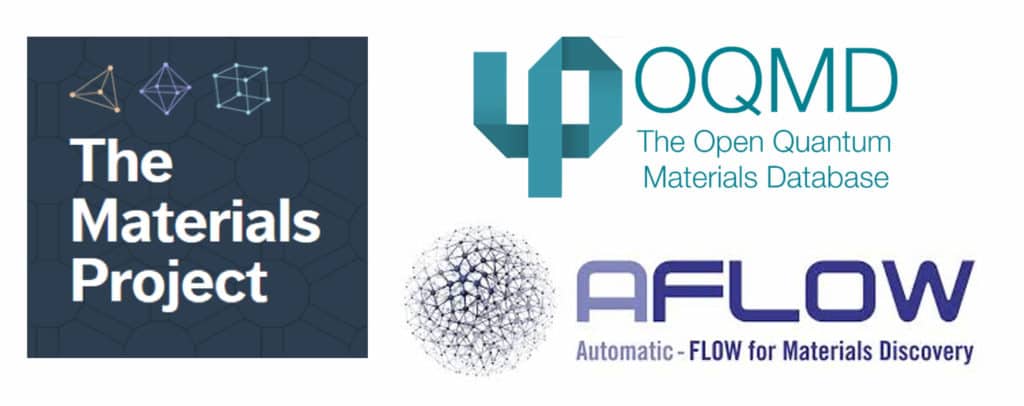
But what uncertainty surrounds DFT data? And where does it come from?
DFT calculations rely on many different parameters whose values are determined by experts. Choosing different parameters can result in widely different outputs. Understanding how parameter choices affect the uncertainty of the output data will lead to better second generation DFT-based material property databases, and of course, reduce risks in downstream projects.
What has the Citrine team done?
With funding from the US Department of Energy1, the Citrine External Research Department, collaborating with researchers at the Olin College of Engineering and the Molecular Sciences Software Institute, performed and published2 a robust statistical analysis of the data, from three of the largest, open, high-throughput DFT-based material properties databases – Materials Project, AFLOW, and OQMD.
Citrine researchers built an integrated pipeline that:
- Queries and ingests DFT data onto Citrine’s data platform
- Curates and standardizes comparable records across the databases
- Calculates uncertainties and performs root cause analysis to identify the sources of uncertainty
Collaborating Institutions
Why was Citrine in a good position to do this?
Citrine are experts at handling materials data and are often called on to help companies ingest their complex, domain-specific data into our platform. DFT calculations are also commonly used by our customers as part of a digital lab approach, where simulations are used during the initial part of materials development in order to reduce resource-intensive physical experiments. The Citrine team has previously carried out projects using DFT for materials discovery and design.
Case Study: DFT calculations used in Sequential Learning by Panasonic ›
Uncertainty estimation is key to providing materials and chemicals R&D leaders with the information they need to make strategic decisions on research direction. For example, the Citrine Platform provides uncertainty estimates when using machine learning to predict materials properties, and sums the probability of successfully hitting target properties across the design space in order to guide R&D decisions3.
How will this work be used?
The “error bars” in fundamental materials properties resulting from our work can be readily incorporated into multiscale models for improved estimates of design uncertainties and process risks. The team’s analysis on the source of uncertainties provides insights into the relationships between parameter choices and the underlying physical principles and can guide the development of next-generation materials databases.
1 U.S. Department of Energy, Office of Basic Energy Sciences (Award Number DE-SC0015106).
2 A preprint of the full technical paper is available at https://arxiv.org/abs/2007.01988.
3 J Ling, M Hutchinson, E Antono, S Paradiso, and B Meredig. High-Dimensional Materials and Process Optimization using Data-driven Experimental Design with Well-Calibrated Uncertainty Estimates. arXiv preprint arXiv:1704.07423, (2017).